Division of Biostatistics
About the Division
We contribute to the scientific literature on the theory and application of statistical methods for population health data across a wide range of areas. In addition, we collaborate on study design and statistical analysis with other researchers within the Institute and the broader scientific communities. We provide statistical training, teach courses, and mentor students and fellows across the Harvard community.
Research
Research Areas
We develop and aid in the thoughtful application of statistical methods for estimating causal effects of time-varying treatment strategies in observational studies and randomized studies with real-world complications such as treatment nonadherence, loss to follow-up, and competing or truncation events such as death. Our work includes methods that accommodate pragmatic time-varying strategies that may (deterministically or stochastically) depend on time-evolving patient characteristics and can appropriately account for time-varying confounding affected by treatment.
Our research focuses on the development, evaluation, and refinement of statistical methods for the design, monitoring, and analysis of randomized clinical trials, where the unit of randomization may be individual or groups of individuals, to ensure the validity, robustness and efficiency of the trial results. The specific topics include sample size and power calculation methods for designing trials to detect the overall treatment effect and treatment effect heterogeneity, methods for designing and conducting flexible clinical trials with potential early stopping due to efficacy or futility, and methods for analyzing trial results, properly accounting for various forms of missing data.
Our research focuses on developing and applying advanced machine learning and deep learning methods to tackle challenges in analyzing high-dimensional and complex data arising in biomedical and healthcare fields. By leveraging these techniques, we aim to uncover relationships between variables, and improve predictions in diverse areas such as survival analysis, policy evaluation, and longitudinal data analysis.
Our research in Methods for Administrative Databases is dedicated to advancing statistical methodologies for large-scale administrative data sources, such as electronic health records (EHR) and claims databases. We develop and apply privacy-preserving inferential techniques tailored for distributed data networks, facilitating secure multi-site collaborations while protecting patient confidentiality. Methodological innovations include sophisticated longitudinal analysis, interrupted time series design, survival data analysis, and missing data analysis to address complex temporal structures and event dependencies. Our work supports cost-effective, evidence-based decision-making in drug safety and effectiveness, facilitates studies on pharmaceutical use and health outcomes in large populations, and provides robust frameworks that extend the utility of administrative data for scientific and policy-driven insights.
Our research in statistical computing addresses the challenges of analyzing large-scale data, particularly when dealing with multivariate and clustered data where correlations play a key role. By refining methods to handle complex models, such as those found in hospital clusters or longitudinal outcomes, we make data analysis more reliable and efficient. This work supports the practical use of electronic health records (EHRs) and other big data sources in medical research, enhancing the ability to conduct meaningful analyses that inform modern healthcare.
We develop, evaluate, and apply statistical methods for genetics and omics data, including metabolomics, proteomics, methylation, and microbiome. These methods are used to determine and understand the path from gene to disease and the role of the environment using causal inference, mediation analysis, Mendelian randomization, machine learning, and computational statistics. We have developed and applied these statistical genetics and genomics methods to a diverse range of traits including depression, anxiety, asthma, COPD, cigarette smoking, cardiovascular disease, HIV, AIDS, Alzheimer’s disease, sleep measurements, and maternal health.
Research Projects
Who We Are
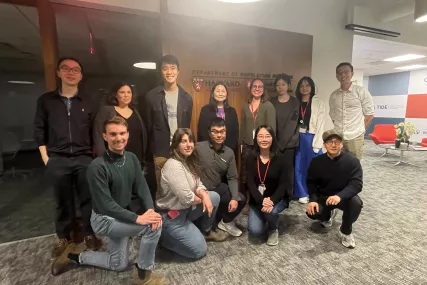